A pioneering researcher and the so-called "Godfather of AI" Geoffrey Hinton resigned from Google to speak more freely about the dangers of the technology he helped create.
During his decades-long career, Hinton's pioneering work in deep learning and neural networks laid the foundation for much of today's AI technology.
Some of the dangers of AI chatbots are "pretty scary", Hinton told the BBC. "At the moment they are no more intelligent than us, as far as I can tell. But I think they could be soon."
In an interview with MIT Technology Review, Hinton also pointed to "bad actors" who could use AI in ways that could have harmful consequences for society - such as manipulating elections or inciting violence.
At the heart of the debate about the state of AI is whether the greatest dangers lie in the future or in the present. On one side are hypothetical scenarios of existential risks caused by computers surpassing human intelligence. On the other side are concerns about automated technology that is already widely deployed by companies and governments and could cause real-world harm.
"For better or for worse, what the chatbot moment has done is turn AI into a national conversation and an international conversation that is not just made up of AI experts and developers," said Alondra Nelson, who until February headed the White House Office of Science and Technology Policy and drafted guidelines around the responsible use of AI tools.
A number of AI researchers have long been concerned about race, gender and other forms of bias in AI systems, including text-based large language models trained on large amounts of human texts, which can reinforce discrimination in society.
"We need to step back and really think about whose needs are central to the discussion of risk," said Sarah Myers West, director of the non-profit AI Now Institute. "The damage done by AI systems today is really not evenly distributed. It exacerbates existing patterns of inequality."
Hinton was one of three AI pioneers who won the 2019 Turing Award, an honour that has become known as tech industry's version of the Nobel Prize. The other two winners, Yoshua Bengio and Yann LeCun, have also expressed concerns about the future of AI.
Many think AI is something new but it is not. AI has been around for a very long time.
From 1957 to 1974, AI flourished. Computers could store more information and became faster, cheaper, and more accessible. Machine learning algorithms also improved and people got better at knowing which algorithm to apply to their problem. Early demonstrations such as Newell and Simon’s General Problem Solver and Joseph Weizenbaum’s ELIZA showed promise toward the goals of problem solving and the interpretation of spoken language respectively. These successes, as well as the advocacy of leading researchers (namely the attendees of the DSRPAI) convinced government agencies such as the Defense Advanced Research Projects Agency (DARPA) to fund AI research at several institutions. The government was particularly interested in a machine that could transcribe and translate spoken language as well as high throughput data processing. Optimism was high and expectations were even higher. In 1970 Marvin Minsky told Life Magazine, “from three to eight years we will have a machine with the general intelligence of an average human being.” However, while the basic proof of principle was there, there was still a long way to go before the end goals of natural language processing, abstract thinking, and self-recognition could be achieved.
In the 1980’s, AI was reignited by two sources: an expansion of the algorithmic toolkit, and a boost of funds. John Hopfield and David Rumelhart popularized “deep learning” techniques which allowed computers to learn using experience. On the other hand Edward Feigenbaum introduced expert systems which mimicked the decision making process of a human expert. The program would ask an expert in a field how to respond in a given situation, and once this was learned for virtually every situation, non-experts could receive advice from that program. Expert systems were widely used in industries. The Japanese government heavily funded expert systems and other AI related endeavors as part of their Fifth Generation Computer Project (FGCP). From 1982-1990, they invested $400 million dollars with the goals of revolutionizing computer processing, implementing logic programming, and improving artificial intelligence. Unfortunately, most of the ambitious goals were not met. However, it could be argued that the indirect effects of the FGCP inspired a talented young generation of engineers and scientists. Regardless, funding of the FGCP ceased, and AI fell out of the limelight.
Ironically, in the absence of government funding and public hype, AI thrived. During the 1990s and 2000s, many of the landmark goals of artificial intelligence had been achieved. In 1997, reigning world chess champion and grand master Gary Kasparov was defeated by IBM’s Deep Blue, a chess playing computer program. This highly publicized match was the first time a reigning world chess champion loss to a computer and served as a huge step towards an artificially intelligent decision making program. In the same year, speech recognition software, developed by Dragon Systems, was implemented on Windows. This was another great step forward but in the direction of the spoken language interpretation endeavor. It seemed that there wasn’t a problem machines couldn’t handle. Even human emotion was fair game as evidenced by Kismet, a robot developed by Cynthia Breazeal that could recognize and display emotions.
We now live in the age of “big data,” an age in which we have the capacity to collect huge sums of information too cumbersome for a person to process. The application of artificial intelligence in this regard has already been quite fruitful in several industries such as technology, banking, marketing, and entertainment. We’ve seen that even if algorithms don’t improve much, big data and massive computing simply allow artificial intelligence to learn through brute force. There may be evidence that Moore’s law is slowing down a tad, but the increase in data certainly hasn’t lost any momentum. Breakthroughs in computer science, mathematics, or neuroscience all serve as potential outs through the ceiling of Moore’s Law.
This immediately answers the question of whether and since when elections can be rigged.
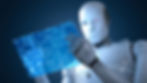